Benchmarking Performance of Different Deep Nude Algorithms
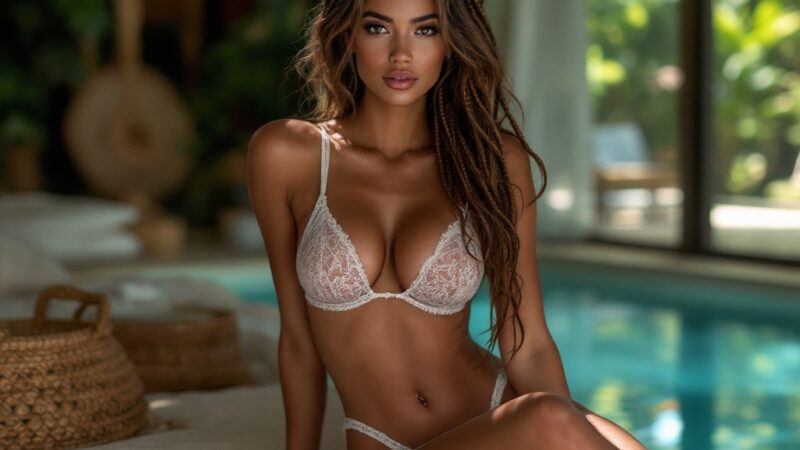
In our current era of swift technological progress, deep nude algorithms have become both a topic of intrigue and concern. These algorithms harness artificial intelligence to produce deepfake imagery, a concept attracting significant attention as it transforms various sectors, particularly the adult entertainment field. The allure is in their knack for crafting extraordinarily lifelike images that blur the boundary between reality and fantasy. As we traverse this complex domain, it becomes vitally important to benchmark these algorithms with precision. The aim is to maintain high quality, uphold ethical standards, and enhance user experiences amidst the growing worries about their application.
Gaining insight into how to effectively assess deep nude algorithms is crucial for both developers and users. Benchmarking offers a framework for precise performance evaluations, highlighting the strengths and pitfalls of different models. In a landscape rich with innovation, the strategic assessment’s importance cannot be overstated. It facilitates the discovery of the most appropriate algorithms for varying uses, paving the way for advancements that genuinely prioritize user satisfaction and ethical obligations. We will explore a range of notable deep nude algorithms, contrasting their performance through meticulous benchmarking.
Grasping AI Benchmarking
Fundamentally, benchmarking is a method of comparative analysis aimed at evaluating algorithms and systems within the realm of artificial intelligence. This involves assessing performance against predefined metrics that carry significance in this field. The process encompasses various aspects, such as accuracy, processing speed, integration with current frameworks, and resilience against misuse. Within the context of deep nude algorithms, proficient benchmarking can lead to revolutionary progress, ensuring that creators adhere to standards focusing on ethical concerns while delivering impactful results. This way, innovation can be appreciated while maintaining a vigilant stance on its consequences.
An Overview of Various Deep Nude Algorithms
Diving into the nuts and bolts, several key algorithms shape the landscape of deep nude technology. Each offers distinct characteristics contributing to their performance and applications. Some of the most prominent include:
- GANs (Generative Adversarial Networks): Renowned for generating high-quality imagery, GANs involve two neural networks challenging each other, continuously honing their outputs.
- VAEs (Variational Autoencoders): These algorithms are adept at image reconstruction, allowing for diverse stylistic variations while keeping essential features intact.
- RNNs (Recurrent Neural Networks): Often used for sequential data, RNNs can create images using contextual clues, thus enhancing narrative coherence.
Benchmarking Methodology
To proficiently evaluate these algorithms, a thorough methodology is indispensable. This procedure starts with selecting diverse metrics to be examined during the benchmarking phase.
The following metrics are commonly considered:
- **Accuracy:** The degree to which the generated images resemble actual photographs.
- **Speed:** The duration required by the algorithm to produce an image.
- **Fidelity:** The level of detail and quality in the synthetic images.
Furthermore, a standardized approach for data acquisition and analysis ensures consistency, reducing biases and enhancing result reliability. This systematic approach establishes a solid base for meaningful comparisons, guiding the future development of these technologies.
Algorithm Type | Strengths | Weaknesses |
---|---|---|
GANs | Impeccable image quality, highly adaptable | Challenging to train, may be unstable |
VAEs | Excellent at feature discovery, more manageable training | Lower fidelity images compared to GANs |
RNNs | Excels with sequences, strong contextual insight | Slower to process, intricate architecture |
Performance Comparison of Algorithms
Following an exhaustive evaluation using the specified metrics, the comparative analysis of these algorithms uncovers fascinating insights. For example, although GANs are frequently praised for their image quality, their training complexity can be a hurdle. In contrast, VAEs offer a more straightforward approach, though with potential compromises in fidelity. While traditionally not the go-to for image creation, RNNs offer distinct benefits in contexts requiring intensive narratives.
Practical Applications of Deep Nude Algorithms
The scope of deep nude algorithms extends deeply into the adult industry, where their realism intrigues viewers and meets various business requirements. They revolutionize consumer interactions by enhancing video content or developing realistic avatars for adult entertainment platforms. However, with these capabilities come serious ethical concerns that cannot be brushed aside. Stakeholders must remain alert to the risk of misuse, ensuring that both creators and consumers are shielded. Ethical considerations regarding consent, authenticity, and privacy must be woven into the discourse.
Conclusion
By surveying the landscape of deep nude algorithms, it becomes clear that benchmarking is integral to refining their effectiveness and usage. Through rigorous methodologies, stakeholders can identify the most effective technologies while considering ethical implications. As we look forward, maintaining a balance between innovation and responsibility will chart the course for AI technologies within the adult industry. Observing the evolution of these algorithms inspires the imagination and spurs essential discussions about the future of content creation.
FAQ
- What are deep nude algorithms?
Deep nude algorithms are artificial intelligence tools designed to create convincingly realistic deepfake images, especially in adult content. - Why is benchmarking vital for these algorithms?
Benchmarking allows developers to assess and compare the performance and efficacy of different algorithms, ensuring the best results. - What ethical issues surround deep nude algorithms?
Ethical challenges include issues of consent, the misuse of technology, and potential harm to individuals’ privacy and reputation. - How can these algorithms be enhanced?
Ongoing research and development that focus on ethical standards, better image quality, and user protection can advance these algorithms. - Are there legal considerations for using deep nude algorithms?
Yes, there are potential legal issues related to copyright, consent, and deepfake content distribution. Always consider the legal framework before using these technologies.
Comments are closed.