Mitigating Mode Collapse in GANs Trained on Erotic Datasets
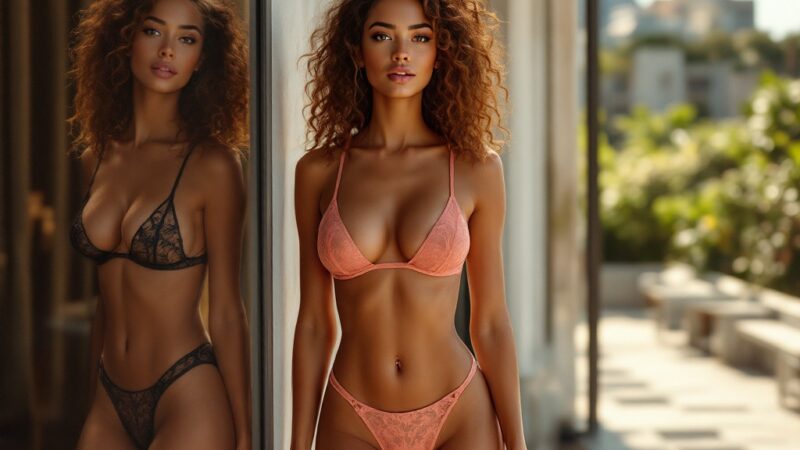
In the ever-evolving landscape of artificial intelligence, there exists a fascinating convergence where creativity intertwines with technology, particularly in the creation of erotic content. At the forefront of this innovation are Generative Adversarial Networks (GANs), robust algorithms that can produce captivating and diverse results. Nonetheless, when these networks are applied to erotic datasets, they encounter a significant hurdle known as mode collapse, which results in repetitive outputs lacking in variety. This article explores the complexities of addressing mode collapse in GANs trained on erotic datasets, offering valuable insights and practical strategies for professionals. By carefully examining both theoretical and practical dimensions of GANs, our aim is to foster a profound understanding and enhance the generation of machine-produced erotic content.
GANs show remarkable prowess in crafting intricate images and videos; however, erotic datasets present unique challenges. As we traverse this specialized terrain, it’s crucial to consider the ethical implications and societal expectations tied to erotic content. To fully exploit the potential of GANs, it is essential that generated outputs mirror the diversity found within the source datasets, thereby not only elevating the content’s quality but also broadening its representation of erotic themes.
Understanding Generative Adversarial Networks (GANs)
Generative Adversarial Networks are composed of two main entities: the generator and the discriminator. The generator crafts new data points, aiming to closely mimic the original training data, while the discriminator assesses the authenticity of this novel data. These two components engage in an ongoing competitive dynamic in which the generator continually seeks to outsmart the discriminator. Through this adversarial training, over time, GANs achieve the capability to create astoundingly realistic outputs across varied areas such as imagery and video. Their ability to assimilate complex data distributions makes them particularly suitable for creative endeavors.
Yet, the generation of erotic content introduces additional layers of complexity. It’s crucial to pay attention to the dataset’s makeup and the ethical consequences of the resulting materials. Without diverse training sets, a GAN risks perpetuating stereotypes and producing lackluster, monotonous results, thereby compromising its effectiveness. Therefore, gaining a comprehensive understanding of GAN operations and the nuances of the datasets they are trained on is imperative, especially in the context of erotic content.
The Importance of Diversity in Erotic Datasets
Diversity is absolutely critical when building generative models for erotic content. A diverse dataset captures a wide array of themes, body types, and expressions, reflecting the spectrum present in the general populace. This variety leads to richer and more multifaceted outputs, enhancing both user interaction and satisfaction. When a GAN is restricted to a uniform dataset, the consequence can be a generation of content that lacks representation and alienates the audience.
In considering the challenges posed by erotic data, a few factors come into play:
- The imperative of ethical portrayal, steering clear of damaging stereotypes.
- How societal norms shape the acceptability of the generated content.
- The danger of losing artistic subtleties due to repetitive outputs.
By addressing these challenges, content creators can maintain the integrity and richness of the outputs generated by GANs in this domain.
The Unique Challenges of Erotic Data
While there are tremendous advantages to deploying GANs for erotic content creation, the unique characteristics of erotic data introduce specific complexities. A major challenge is to retain control over the content to ensure adherence to ethical standards. A thorough understanding of diverse human experiences is essential, as a narrow focus might oversimplify intricate narratives. Additionally, the emotional and psychological ramifications of erotic content exert intrinsic pressure on creators to balance between artistic expression and its societal implications.
Given these hurdles, strategies must be applied to ensure GAN training on erotic datasets remains effective. It is imperative to adopt a thoughtful approach to dataset curation and refinement, alongside a genuine commitment to upholding ethical considerations.
Mode Collapse: An Overview
Mode collapse arises when a GAN churns out a very limited variety of outputs, choosing a few modes of the dataset rather than fully exploring the spectrum of possible outputs. This phenomenon is especially challenging in generating diverse erotic content, where different themes and styles are crucial to keep things engaging. The presence of mode collapse stifles the creative potential of GANs, leading to diminished user engagement and satisfaction.
Indicators of mode collapse in the creation of erotic content include:
- Uniformity in outputs, with many images appearing strikingly similar.
- A lack of thematic variation, resulting in the repetition of styles or motifs.
- Inadequate representation of training dataset diversity, such as different body types.
Signs of Mode Collapse in Erotic Content Generation
Creators and users must stay alert for the signs of mode collapse. Ignoring it can lead to unchanging results and minimal audience engagement. While some consistency in output is desirable, excessive sameness indicates an underlying issue with the model’s capacity to generate diversity.
Indicator | Description |
---|---|
Uniform Outputs | Reoccurring or nearly identical images produced by the GAN. |
Thematic Monotony | Limited variety in themes creates a lack of diverse content. |
Inadequate Diversity Reflection | Failure to mirror the full spectrum of the original dataset. |
Strategies for Mitigating Mode Collapse
Addressing mode collapse efficiently requires employing multiple strategies when working with GANs trained on erotic datasets. These approaches not only foster diversity but also boost the model’s overall efficacy. A comprehensive methodology, involving a collection of techniques, is recommended.
Data Augmentation Techniques
Data augmentation is crucial in thwarting mode collapse by injecting variability into the dataset. Methods like rotation, resizing, and color adjustments allow the model to encounter diverse manifestations of the same foundational data. Implementing data augmentation can significantly enrich the input data’s complexity, thus enhancing the final outputs.
Effective data augmentation techniques include:
- Rotating images horizontally or vertically.
- Applying random cropping to the images.
- Varying brightness or contrast to introduce elements of unpredictability.
Implementing Diverse Training Objectives
Besides data augmentation, introducing varied training objectives and constraints during model training can elevate the diversity of created outputs. Establishing multiple goals and challenges for the generator encourages the GAN to explore different dimensions of the dataset. This strategy, which can elevate creativity within the model, leads to richer outcomes and a more captivating user experience. It can involve techniques such as:
- Employing multi-task learning techniques.
- Integrating adversarial training processes focused on distinct elements.
- Using loss functions that explicitly promote diversity.
Architectural Adjustments and Innovations
Finally, revolutionary architectural changes can assist in minimizing mode collapse within GANs. Enhancing both the generator’s and discriminator’s architecture can foster a more varied output landscape. Options include employing advanced architectures like Conditional GANs (cGANs) or Progressive Growing GANs (PGGANs), which naturally induce results with more variance. By seamlessly integrating these architectural tactics, practitioners can lead their models toward producing a wider array of erotic content.
Regular Evaluation and Monitoring
The continual evaluation and observation of GAN performance are critical to ensuring output diversity during the training process. Constant assessment helps practitioners detect early signs of mode collapse, allowing for the adjustment of strategies accordingly. By applying robust evaluation protocols and leveraging feedback from generated content, models can remain flexible and responsive to the challenges presented by erotic datasets.
This approach involves frequent examination and fine-tuning of GAN parameters, as well as gathering user feedback on generated content. Establishing clear success metrics will further reinforce the model’s ability to deliver varied and engaging outputs.
Conclusion
Tackling mode collapse in GANs trained on erotic datasets presents a multifaceted challenge requiring both thoughtful approaches to dataset diversity and pioneering technological adjustments. By understanding GAN fundamentals, considering the specific challenges posed by erotic datasets, and adopting a range of tactics from data augmentation to architectural innovations, practitioners can improve the quality and intricacy of machine-generated content. Pursuing variety not only addresses developers’ creative ambitions but also enriches the user experience, fostering deeper connections with audiences.
FAQ
- What is mode collapse in GANs?
Mode collapse is when the generator creates minimal variations, leading to a lack of diversity in outputs. - How does mode collapse affect the generation of erotic content?
It can produce repetitive or monotonous outputs that fail to represent the dataset’s diversity accurately. - What role does data augmentation play in addressing mode collapse?
Data augmentation introduces variability in the dataset, making it challenging for the model to focus on a single output, thus promoting diversity. - Are there ethical considerations when training GANs on erotic datasets?
Yes, ethical considerations involve ensuring suitable representation and avoiding perpetuating harmful stereotypes. - Can architectural changes really mitigate mode collapse?
Indeed, innovative architectural adjustments can promote a greater diversity in outputs and lessen the risk of mode collapse.
Comments are closed.