Public Health Surveillance Enhanced by Data-Driven Models
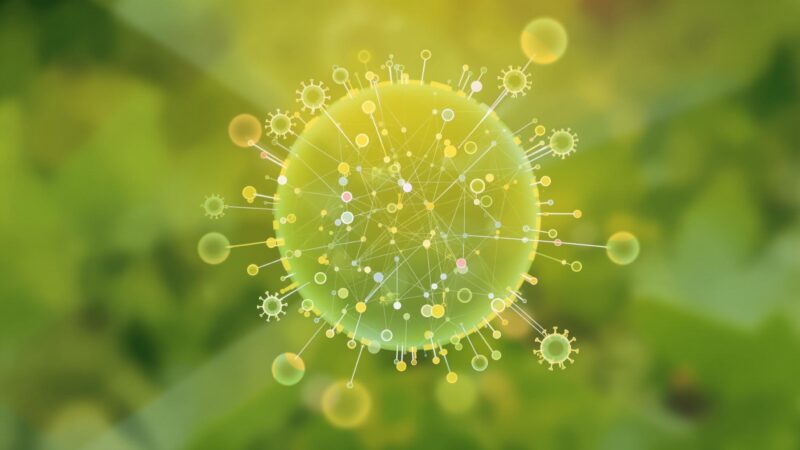
Deploying technology alongside public health creates a fresh approach for attending to health issues for which data has become central. Watching a particular phenomenon closely, especially when dealing with prevention and control of diseases, has a technique called Satellite modalities which does not require direct supervision (means public health supervision) and is grounded on logic that is data-centered and needs-based. To put this bluntly, these programs, no matter how complex they might look, allow authorities to sweep through intricate data and devise algorithms which tell them what is likely to happen in the future, so that they can plan accordingly. Regardless of the circumstances, it is important to mention that the contribution of worsening health issues changes value to cost in relevance. In this particular lesson, we will look at these public health surveillance models and their potential impact in the future.
By taking this approach, communities will be able to target their healthcare efforts to be more effective, save lives, and reduce the cost to the community. These firms use the advanced algorithms, statistics, and automated machine learning programs which are collectively called “data-driven models” to make sense of information that was previously useless. There is now a shift from programed based health surveillance to data driven public health surveillance which changes how the authorities and the health institutions deal with the health of the population. Intelligent modeling brings data to life and makes it possible to proactively address health emergencies instead of waiting for health crises to occur.
The Role of Data-Driven Models in Public Health
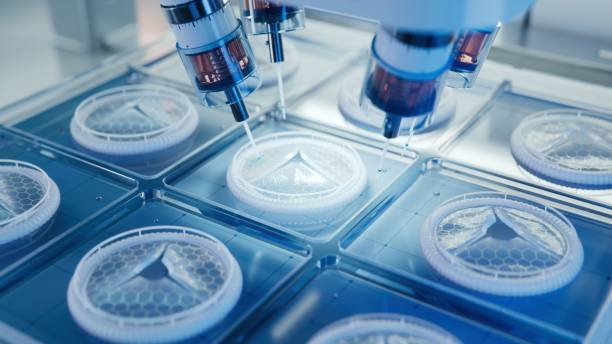
The data-driven model utilizes quantitative information to analyzes trends in health and is single-handedly the most advanced paradigm in the field of public health surveillance. These models utilize predctive algorithms, which merge historical data with real-time information to create futurictic scenarios.
Usinig realtime data such as the epidemiological state along with historical data, these models have the capability of forecasting an outbreak of an infectious disease like influenza. Predictive Models. Spatial Analysis Models These geographic focus models make it possible to display data panels where there are health problem so that intervention can be directed to the areas with greater needs.
These models enable evidence-based reasoning about the effects of different health program interventions by predicting outcomes for various intervention choices. Simulation Models.
Benefits of Enhanced Surveillance through Data-Driven Models
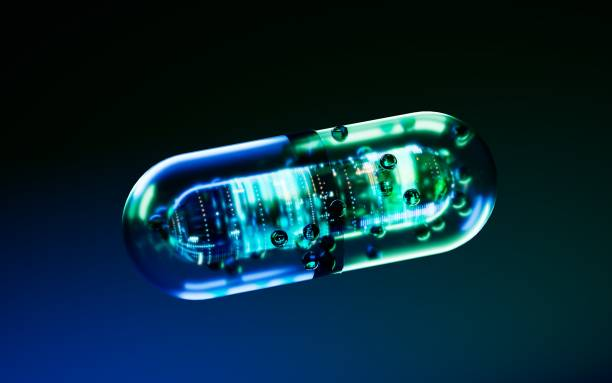
Integrating data-driven approaches in public health surveillance has many advantages that result in positive health outcomes. These benefits include improved accuracy in the gathering of health information which is vital in formulating effective public health policies.. Moreover, clear analytics allow public health officials to distill relevant signals amid noise, improving insights from the data.
- Improved Accuracy of Data: Large volumes of data enhance the precision of health assessments.
- Timely Response to Health Threats: Predictive models allow quicker reactions to outbreaks, reducing their spread.
- Resource Optimization: Data-driven insights ensure that interventions are targeted where they are needed most.
Here is a brief overview of the benefits offered by data-driven models in public health surveillance:
Benefit | Description |
---|---|
Improved Accuracy | Reduces errors by utilizing comprehensive datasets. |
Proactive Measures | Allows for forecasts of outbreaks before they escalate. |
Efficient Resource Allocation | Directs resources to areas of greatest need based on real-time data. |
Challenges in Implementing Data-Driven Models
Even though they could bring about change, the implementation of data driven models for public health surveillance systems comes with a lot of drawbacks. One of the foremost challenges is the data security issue, particularly the gathering and analysis of health data which is very private. Authorities need to ensure that there is enough value to be gained from the consolidated data while protecting privacy for personal information.
In addition to these, the concern of quality for the data cannot go unaddressed. The models trust the information fed into them and that trust is entirely baseless. If the data is poor, public health response systems will suffer because these systems will draw incorrect conclusions. As health data becomes more intricate, it becomes increasingly difficult, yet more vital, to guarantee that what is being collected is valuable and precise for health management purposes.
Future Directions in Public Health Surveillance
In the realm of public health surveillance, the evolution is quite refreshing. The progress of technology, artificial intelligence, and machine learning will be an asset to data-based models. AI technology can assist in realtime health surveillance effectively because it processes massive amounts of data at a far quicker speed than the traditional methods.
- Integration of Artificial Intelligence: AI is set to bolster predictive models, improving accuracy and efficiency in data analysis.
- Collaboration Across Disciplines: Increased collaboration between public health officials, data scientists, and technologists will further enhance surveillance efforts.
- Telehealth Data Utilization: Expanding the use of telehealth data can provide a more holistic view of public health trends.
Conclusion
As highlighted, data-driven models are not an enhancement in public health surveillance; they are revolutionary. The ability to gather, analyze, and apply massive amounts of health data allows officials in public health to solve problems more effectively. These models have just begun to show the world what can be achieved with precision, prompt action and improved resource allocation. Every day the world of health becomes more complex and solving these challenges will need to be done. The onus is on us to take action as the future of public health surveillance will depend on how these advancements are addressed.
Frequently Asked Questions
- What is public health surveillance? Public health surveillance is the systematic collection and analysis of health-related data to monitor and prevent diseases.
- How do data-driven models enhance public health surveillance? They improve the accuracy, speed, and overall effectiveness of tracking diseases and health trends.
- What are the common types of data-driven models used in public health? Predictive models, spatial analysis models, and simulation models are commonly used.
- What challenges do data-driven models face in public health? Challenges include data privacy concerns and issues related to the quality and accuracy of the data collected.
- What is the future of public health surveillance? The future includes the integration of artificial intelligence and strengthening collaborations across various disciplines.
Comments are closed.