Public Safety Reinvented: The Role of Predictive Analytics
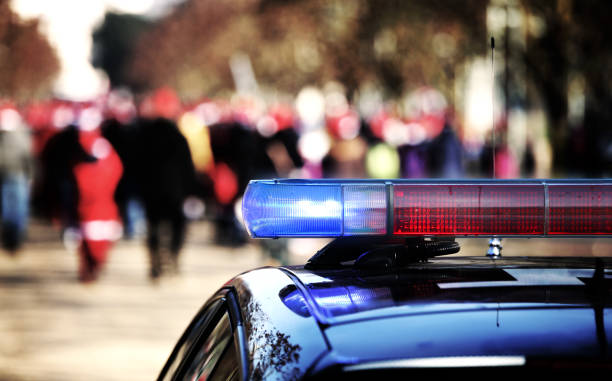
The use of predictive analytics is transforming the field of public safety. Such a strategy uses large databases of information to foretell a certain event, in this case, aiding enforcement officers and emergency managers in their decision making. Instead of remaining bound to of the old mindset of reactive response to problem, agencies have now acquired the capability to proactively prevent issues from arising. The more advanced the public spaces in a given city are, the more difficult it is to ensure safety, and with that challenge, governance becomes increasingly important to predictive analytics. These systems can identify automated patterns that a human is most likely to overlook with the help of historical data and complex algorithms. This paves the way for smarter resource allocation, enhanced citizens – authorities relations and community engagement.
Understanding Predictive Analytics
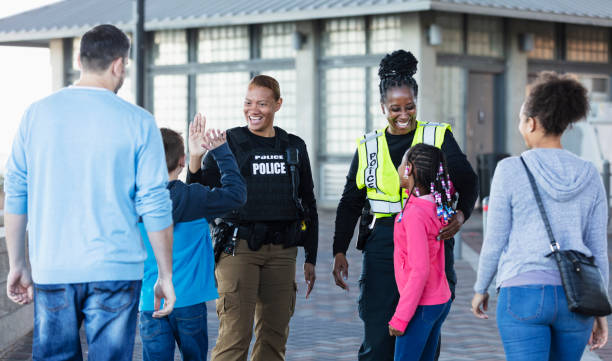
Predictive analysis is first blending prognosis and insights analysis with performance estimation. Predictive analytics employs a blend of techniques such as statistics, data mining, and machine learning to analyze past performance of an entity and predicts future results. The predictive intelligence endeavor is a programmatic and systematic blending of processes and components such that data is converted into information and then into insight. Describing these components will surely help discuss the promises that predictive analytics that pertain to transformation of public safety. The reason why these algorithms which do the mathematical executions perform more than mere equations can be put in the context of behavior and socio-ecological intelligence enables good decision making. Thus, within the context of policy and management of public safety issues, the importance and relevance of predictive analysis cannot be understated.
A working understanding of predictive analytics requires knowledge of its framing parts. Predictive analytics incorporates techniques like data collection, data analysis, and machine learning, making it highly effective. Collecting data happens when the relevant information is captured from the past, which is where the analytics come from. The rest is known as the analysis phase, which defines patterns within the data that the organizations would incorporate to understand the data. Following that, an algorithm adapts to incoming data over time and becomes more accurate at predicting responses. Any public safety solution becomes more precise as there is better refinement of the algorithm and its ability to deal with issues as they occur.
The Importance of Data in Predictive Analytics
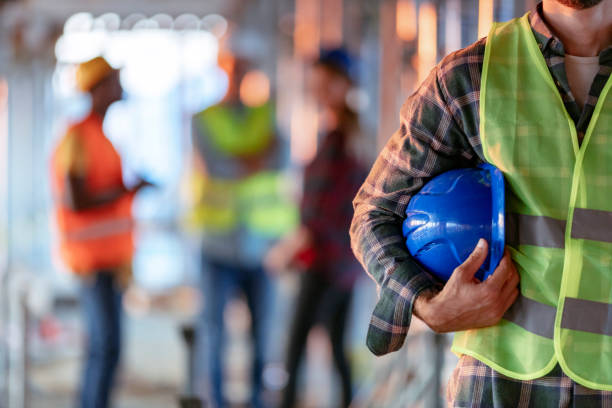
For public’s security, data translates to vital information regarding trends and is useful for analytics of violence, making it the most crucial aspect. Potential data sources can include everything from weather patterns and social media usage to reports on crime and population details. Considering this, agencies are capable of identifying and forecasting violence scenarios in detail, deploying their resources appropriately to manage crises before they escalate. Predictive analytics is only as good wishes as the accuracy and authenticity of the information provided. Quality information gives reliability in predictions, whereas poor quality results in predictions that leads to waste and large amounts of plummeting resources.
In terms of aiding predictive analytics for violence, the following would assist:
Compilation of social media posts to track trends, attitudes and phenomena within the community.
A mixed approach involving all kinds of information forms a complex tapestry of the public safety problems that exist. This allows agencies to operate in dynamic settings and ensures that attention is relevant and offered in a timely manner. However, as data becomes more accessible, there will be a need for concentration on the quality and credibility of the information that is to be utilized.
Data Type | Purpose | Example |
---|---|---|
Historical Crime Data | Identify crime patterns | Frequency of thefts in a neighborhood |
Social Media Activity | Gauge community sentiment | Posts discussing recent disturbances |
Environmental Data | Understand influencing factors | Weather conditions during crime spikes |
Well-informed safety officials take full advantage of everything that predictive analytics has to offer to make discerning decisions. Successful implementation, however, poses challenges that must be resolved.
Applications of Predictive Analytics in Public Safety
For now, I will use some illustrative examples, which I think many will agree, can be technology-assisted public safety challenges. I could mention law enforcement efforts to suppress crime, containing hotspots where crime is predicted to occur, and prevent it from occurring. Clearly, placing resources into the right zones is aided by crime prediction analytics that identify such ‘hotspot’ zones. Moreover, drastic optimization of resource allocations can also be achieved with other types of emergency response predictive analysis, where resource allocation decisions are based on learned incidents that allow for more accurate positioning of responder teams. Modern tools also allow for efficient risk evaluation and risk monitoring, enabling agencies to plan strategic measures early enough to prevent relevant crises from occurring.
Rather than simply responding to conflicts after they occur, these new systems allow police to be proactive. Predictive modeling based on previously completed values helps determine where crime is likely to happen, facilitating focused policing and community visibility. To conclude, the optimization of predictive analytics increases individual security as well as places the police ahead of the offenders.
Barriers to Predictive Analysis Implementation
The integration of predictive analytics into systems for public safety possess a myriad of problems. The first concern is in the realm of ethics. There is skepticism regarding whether the data has been sanitized in such a way that some populations are, more often than not, filtered out. Ethically, there is the overarching concern of the massive amount of private information that is collected and processed. On top of this, another considerable obstacle is infrastructure. Many public agencies are suffering through the stagnation of the old systems that cannot keep up with the sophisticated analytics that need to be performed. They end up needing to invest in technology which a lot of public safety agencies of seem to have, thus expanding the implementation gap even further.
Conclusion
Predictive analytics and public safety is a synergetic space that demonstrates how diverse disciplines are being intertwined and the impact of technology on what’s achievable towards improving performance. Law enforcement can evaluate available data to decrease the chances of a crime occurring, optimizethe use of each resource, and strengthen community safety. Despite the advantages, this change poses particular challenges such as ethical issues and insufficient technological development. With a shift in mentality, the adoption of these broad tools provides a canvas for imagining a public safety future with extraordinary enhancements. Essentially, modern communities that intend to meet the changing times and provide comprehensive protection to its residents need to utilize predictive analytics.
Frequently Asked Questions
- What is predictive analytics? Predictive analytics is a branch of advanced analytics that uses historical data, statistical algorithms, and machine learning techniques to identify the likelihood of future outcomes.
- How is predictive analytics used in public safety? It is used to forecast crime patterns, optimize emergency responses, and assess risks within communities.
- What types of data are involved in predictive analytics? Common types of data include historical crime records, demographic information, social media activity, and environmental factors.
- What are the ethical concerns surrounding predictive analytics in public safety? Concerns include potential biases in data leading to unfair targeting of certain demographics and privacy issues related to data collection and usage.
- What technological advancements are shaping the future of predictive analytics? Innovations such as real-time data processing, enhanced machine learning algorithms, and improved data visualization techniques are paving the way for more effective predictive analytics.
Comments are closed.